个人信息
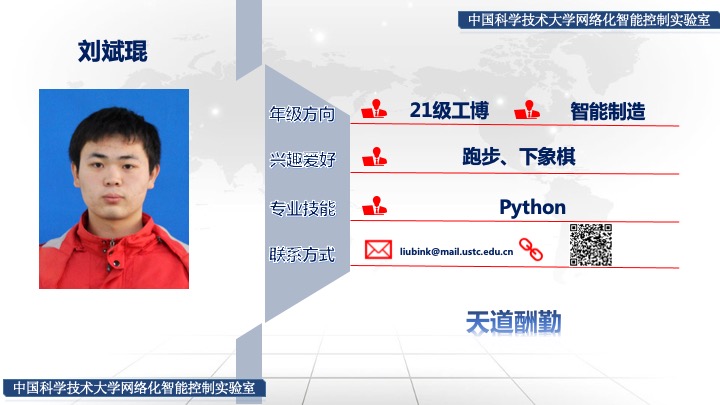
参与实验室科研项目
人机智能协同关键技术及其在智能制造中的应用
掘进机盾尾密封及刀具状态智能诊断与评价技术研究
非可信智能驱动的可靠智造
学术成果
共撰写/参与撰写专利 10 项,录用/发表论文 4 篇,投出待录用论文3篇。
patent
-
眼科病人信息录入软件V1.0
康宇,
田霞,
董凯,
鲁理,
夏睿钰,
赵云波,
刘斌琨,
and 李晓蒙
2023
[pdf]
-
锡膏印刷机参数调整数据处理软件V1.0
许镇义,
刘斌琨,
康宇,
曹洋,
and 赵云波
2022
[pdf]
-
锡膏印刷机离线故障预测软件V1.0
赵云波,
刘斌琨,
曹洋,
康宇,
and 许镇义
2022
[pdf]
-
基于特征工程的PCB板焊盘偏移预测方法及存储介质
曹洋,
刘斌琨,
赵云波,
康宇,
and 许镇义
[Abs]
本发明的一种基于特征工程的PCB板焊盘偏 移预测方法及存储介质,包括以下步骤,S1:获取 PCB板的历史偏移检测数据,进行数据预处理,并 使 用滑动窗 格法对数据进行 切分 ;S2 :计算手工 设计的统计指标;S3:构建重加权支持向量机,输 出预测结果。本发明可根据手工设计的反应偏移 时间序列特点的统计特征对PCB板焊盘偏移进行 预测,通过工程师的适时介入从而避免生产不良 品 。同 时 可通过统 计特征对预 测结果进行解 释 , 使得模型预测过程更容易被工程师理解。利用加 权的支持向量机对手工设计的特征进行核函数 的 非线性变换 ,提高 模型的 稳定性。对偏移不良 赋予更大的权重,克服了偏移不良数据极其稀少 的困难,从而实现偏移不良的时序预测。
-
一种基于多传感器特征融合的轴承故障诊断方法
康宇,
刘斌琨,
赵云波,
许镇义,
and 曹洋
-
一种基于时间重构图卷积的PCB锡膏印刷质量预测方法
康宇,
刘斌琨,
赵云波,
曹洋,
许镇义,
and 柏鹏
[Abs]
本发明公开了一种基于时间重构图卷积的 PCB锡膏印刷质量预测方法,包括获取PCB板的历 史锡膏体积检测序列和对应的检测时间的历史 数据,并进行预处理;建立时间重构模块,根据对 应的检测时间的时间间隔对历史数据进行重构, 得到重构数据;建立时空图卷积模块,提取得到 的重构数据的锡膏体积之间的关联性和锡膏体 积自身的变化规律;根据锡膏体积之间的关联性 和锡膏体积自身的变化规律构建线性回归器,预 测得到锡膏体积变化规律。本发明根据焊盘上锡 膏体积的时间相关性构建稀疏图以提高相似演 变规律的协同同时避免不同演变规律的干扰然 后根据生产时刻的间隔构建时间注意力对数据 重构后预测PCB锡膏印刷质量。
-
一种基于特征迁移的轴承剩余使用寿命预测方法
许镇义,
刘斌琨,
康宇,
赵云波,
and 曹洋
[Abs]
本发明涉及设备预测性维护技术领域,公开 了一种基于特征迁移的轴承剩余使用寿命预测 方法,包括:获取设备故障数据和设备全周期退 化数据,进行数据预处理;构建源网络,源网络包 括故障特征提取模块、故障分类器;设计故障分 类损失函数,并利用设备故障数据对源网络进行 训练 ;构建目标网络 ,目标网络包括退化特征提 取器和剩余使用寿命预测器;设计剩余使用寿命 预测损失函数,并利用设备全周期退化数据对目 标网络进行训练;设计故障特征迁移损失函数, 进行故障特征迁移。本发明通过对设备进行故障 诊断获取故障特征以及特征迁移,从而降低已有 方法对设备全周期退化数据的需求,并得到较好 的结果。
-
减震器故障检测方法、装置、设备及存储介质
赵云波,
刘斌琨,
康宇,
曹洋,
and 许镇义
[Abs]
本发明涉及设备检测技术领域,公开了一种 减震器故障检测方法、装置、设备及存储介质,该 方法包括:基于待检测减震器的传感器数据获取 正对时频图像和负对时频图像;通过预设编码器 对正对时频图像和负对时频图像进行特征提取, 获得传感器特征集;对传感器特征集中各特征进 行更新,并对更新后的传感器特征集中的特征进 行特征融 合 ,获得融合特征集 ;通过预设线性分 类器对融合特征集进行分类检测,根据分类检测 结果判断待检测减震器是否存在故障。相比于现 有技术中通过花费高额成本来获取大量有标签 数据,本发明上述方法减少了现有技术中对有标 签数据的需求量过大导致成本过高的问题,从而 实现对减震器健康状态的预测性维护检测。
-
故障诊断模型的训练方法、装置、电子设备及存储介质
赵云波,
陈龙鑫,
刘斌琨,
朱慧娟,
许镇义,
and 柏鹏
[Abs]
本申请公开了一种故障诊断模型的训练方 法、装置、电子设备及存储介质,属于计算机技术 领域。所述方法包括:获取多个工况中各工况集 下的故障数据,分别作为源域数据,并获取目标 工况下的故障数据,作为目标域数据,所述工况 集包括所述多个工况中的至少一个工况,所述工 况集下的故障数据为已标记故障类别的数据,所 述目标工况下的故障数据为未标记故障类别的 数据;确定所述目标域数据与各所述源域数据之 间的目标分布差异;根据所述目标分布差异选取 源域数据作为训练数据;根据所述训练数据,对 所述目标工况的故障诊断模型进行训练。本申请 能够提高模型训练效果,进而提高对目标工况进 行故障诊断的准确率。
-
产线设备故障预测方法、装置、电子设备及存储介质
赵云波,
董少杰,
刘斌琨,
朱慧娟,
许镇义,
and 柏鹏
[Abs]
本申请公开了一种产线设备故障预测方法、 装置、电子设备及存储介质,属于计算机技术领 域。所述方法包括:获取测试结果序列,测试结果 序列包括多个电路板的测试结果信息,测试结果 信息包括电路板中各功能模块的测试结果;根据 测试结果序列,获取各功能模块的良率序列;根 据各功能模块的良率序列,获取多个各功能模块 中的目标功能模块与其他功能模块的相关性序 列;根据各功能模块的良率序列和相关性序列, 获取目标功能模块的良率预测信息;根据相关性 序列和良率预测信息,预测目标功能模块对应的 产线设备是否存在故障。本申请能够准确预测产 线设备是否出现故障,以及时发现故障设备,避 免生产事故的发生。
Conference Articles
-
A Reliable Ensemble Model Based on Hierarchical Component Features for Repair Label Prediction of Soldering Defects
Longxin Chen,
Yunbo Zhao,
Binkun Liu,
Shaojie Dong,
Huijuan Zhu,
and Peng Bai
In
2024
[Abs]
[pdf]
Accurate prediction for repair labels of soldering defective printed circuit board (PCB) components can help lower labor costs, using solder paste inspection (SPI) and automated optical inspection (AOI) data. Existing research tries to pick out both the false defect components (actually good) and impossibleto-repair components among defective PCB components, using SPI and AOI data. However, it is inappropriate to pick out the false defect components from screened components using defective information of AOI data. So the problem setting of existing research is inappropriate, resulting in the algorithm’s performance not meeting actual requirements. To address this problem, we only care about the reliable prediction of impossibleto-repair components. We propose a hierarchical component feature extraction method that can comprehensively characterize the degree of component defects from multiple levels, including pin level and component level, and apply ensemble model based on XGBoost and TabNet to the reliable prediction of impossibleto-repair components by adjusting the probability threshold of components judged as impossible-to-repair category. Finally, we validated our method on real datasets and achieved the best experimental results compared to baseline methods, which can meet actual requirements.
-
A Feature Engineering-based Method for PCB Solder Paste Position Offset Prediction
Binkun Liu,
Yunbo Zhao,
Yu Kang,
Yang Cao,
Peng Bai,
and Zhenyi Xu
In 2023 6th Int. Symp. Auton. Syst. ISAS
2023
[Abs]
[doi]
[pdf]
Solder paste printing position offset is a common type of defective printed circuit board (PCB) printing, and accurate position offset prediction helps to avoid the production of defects, thus improving efficiency. The existing methods mainly use the powerful nonlinear fitting ability of deep learning to learn the variation pattern of solder paste printing quality to achieve a good prediction. However, factories also focus on the interpretability of the model, and existing methods are difficult to give the basis for decisions, so there are still limitations in the practical application. To solve this problem, we propose a Support vector machine (SVM) approach, in which we manually design 14 statistical features based on the original data, then the resampling reduces the effect of data imbalance and achieves PCB pad-level offset prediction. Finally we verified on about one week of real solder paste printing production data and achieved good experimental results.
-
A Robustness Benchmark for Prognostics and Health Management
Binkun Liu,
Yun-Bo Zhao ,
Yang Cao,
Yu Kang,
and Zhenyi Xu
In 2022 41st Chin. Control Conf. CCC
2022
[Abs]
[doi]
[pdf]
With the rise of intelligent manufacturing, prognostics and health management(PHM) has developed rapidly as an important part of intelligent manufacturing.Existing deep learning-based PHM methods are data-dependent. However, sensor data often contains noise and is redundant and high-dimensional, making it difficult for the PHM methods to learn a stable set of model parameters, so the methods are likely to be wrong when disturbed. However, the factory hopes that the PHM methods are robust enough to adapt to various disturbances, so it is necessary to perform robustness evaluation on the existing methods in advance for easy deployment. Although the existing robust theoretical analysis methods for neural networks can obtain tight robust boundaries, they consume a lot of computing resources and are difficult to scale to large neural networks. To slove this problem, We design a benchmark for robustness analysis of large deep learning PHM models, in which we test the model robustness using a variety of perturbations to simulate the actual production environment of the factory. Specifically, Gaussian noise is used to test the robustness of the model to background noise; random mask is used to test the robustness of the model to data loss. We hope that our robustness benchmark can serve as a reference for designing PHM models to improve the robustness of factory PHM models.
Journal Articles
-
Cross-Sensor Generative Self-Supervised Learning Network for Fault Detection Under Few Sample
Huijuan Zhu,
Yun-Bo Zhao ,
Xiaohui Yan,
Yu Kang,
and Binkun Liu
J. Syst. Sci. Complex.
2024
[pdf]