个人信息
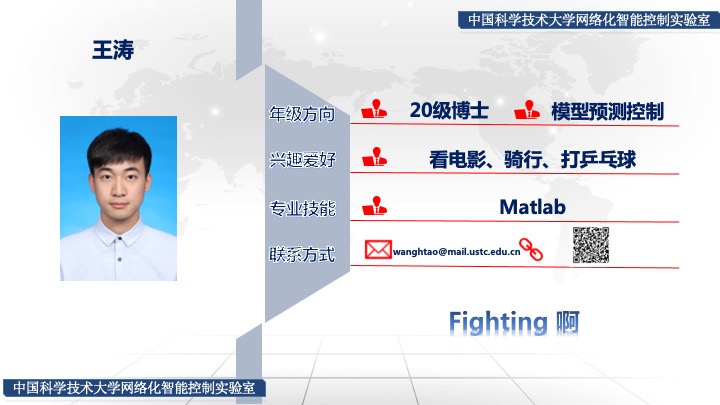
参与实验室科研项目
基于数据驱动和联合设计的无线网络化控制系统的使能建模和设计
研究课题
基于近似模型的模型预测控制、基于事件触发的模型预测控制/分布式模型预测控制
奖励荣誉
2020硕士研究生国家奖学金
学术成果
共撰写/参与撰写专利 3 项,录用/发表论文 12 篇,投出待录用论文1篇。 联培学生可能有其他不在此展示的论文/专利。
patent
-
一种自适应时域的事件触发模型预测控制方法
康宇,
王涛,
李鹏飞,
赵云波,
and 吕文君
2021
[Abs]
[pdf]
本发明的一种自适应时域的事件触发模型 预测控制方法,可解决现有的事件触发的模型预 测控制方法计算负担较大的技术问题。包括根据 实际的被控对象 ,建立系统的离散时间数学模 型 ;根据上述求得的 数学模型 ,定义求解优化问 题的 代价函数 ;定义优化问 题 ,以 及系统的 状态 和控制输入的约束;建立自适应预测时域的数学 表达;求解优化问题得到的预测控制输入和状态 序列构造系统整体的控制输入;控制输入用到系 统后得到的预测状态建立系统的触发条件;根据 上述所有步骤,建立最终用到实际系统的基于自 适应时域的事件触发模型预测控制算法。本发明 预测时域是自适应的 ,随着状态值越靠近终端约 束集,预测时域越小,在线优化计算频率越低,大 大降低计算负担。
-
一种无线云控制系统调度方法及系统
康宇,
李鹏飞,
王涛,
赵云波,
陈绍冯,
and 吕文君
2021
[Abs]
[pdf]
本发明的一种无线云控制系统调度方法及 系统 ,包括根据实际的 各个被控对象 ,分 别建立 系统模型 ;进行云计算平台的 配置 ,确定模型预 测控制方法的预测时域、控制器以及传感器的成 功传输概率需求 ;调整所有传感器的 发送概率 ; 根 据各个传感器的 发送概率 ,确定发 送门 槛 ;根 据是否成功收到各自的传感器数据来计算最优 控制序列,并准备通过控制信道发送给各自的执 行器;中心调度器选择一个控制器来接入控制信 道;各个执行器根据是否成功收到控制序列来更 新缓 冲器内 容 ,并从中 选择控 制量进行执行。本 发明能够使得所有传感器的发送概率达到高效 的 纳什均衡点 ,节约传感器的 发 送功耗 ,避免 控 制信号在控制信道之间的碰撞,并且确保所有控 制系统的稳定性。
-
贴片机的健康度评估方法及系统
李鹏飞,
赵昀昇,
康宇,
赵云波,
and 王涛
[Abs]
本发明公开了一种贴片机的健康度评估方 法及系统,属于工业制造装备技术领域,包括:获 取贴片机当前运行状态数据;采用主成分分析法 对当前运行状态数 据进行特征提取 ,得到i个健 康监 测指标 ;采 用标准化欧氏 距离法 ,根据健康 监测指标在贴片机整体健康情况中的健康权重, 计算各健康监测指标的向量与理想健康向量之 间的距离;采用负向函数将距离换算成贴片机的 健康值。整个预测过程,简单易操作,可解释性很 强,不需要进行复杂地配置,贴合工业环境,无需 占 用过多的计算资源,即实现对贴片机的健康程 度评估。
Journal Articles
-
Rolling Self-Triggered Distributed MPC for Dynamically Coupled Nonlinear Systems
Tao Wang,
Yu Kang,
Pengfei Li,
Yun-Bo Zhao ,
and Hao Tang
Automatica
2024
[Abs]
[doi]
[pdf]
The mutual influences caused by dynamic couplings in large-scale systems increase the difficulty in the design and analysis of distributed model predictive control (DMPC), and require information exchange among subsystems which calls for a scheduling strategy to save communication resources in communication-limited environments. To circumvent the two problems, we design a rolling selftriggered DMPC strategy for large-scale dynamically coupled systems with state and control input constraints. First, the optimal control problem where the cost is subject to the coupled dynamic and the constraints are subject to the uncoupled counterpart is proposed, forming the dual-model DMPC that is simple in design and analysis but yields good control performance. Second, the information exchange only occurs at some specified triggering instants determined by a rolling self-triggered mechanism, saving communication resources more significantly. The effectiveness of the designed strategy is verified by numerical simulations.
-
Compound Event-Triggered Distributed MPC for Coupled Nonlinear Systems
Yu Kang,
Tao Wang,
Pengfei Li,
Zhenyi Xu,
and Yun-Bo Zhao
IEEE Trans. Cybern.
2023
[Abs]
[doi]
[pdf]
This paper investigates the event-triggered distributed model predictive control (DMPC) for perturbed coupled nonlinear systems subject to state and control input constraints. A novel compound event-triggered DMPC strategy, including a compound triggering condition and a new constraint tightening approach, is developed. In this event-triggered strategy, two stability-related conditions are checked in a parallel manner, which relaxes the requirement of the decrease of the Lyapunov function. As a result, the number of triggering instants can be reduced significantly. Furthermore, the proposed constraint tightening approach solves the problem of the state constraint satisfaction, which is quite challenging due to the external disturbances and the mutual influences caused by dynamical coupling. Simulations are conducted at last to validate the effectiveness of the proposed algorithm.
-
Disturbance Prediction-Based Adaptive Event-Triggered Model Predictive Control for Perturbed Nonlinear Systems
Pengfei Li,
Yu Kang,
Tao Wang,
and Yun-Bo Zhao
IEEE Trans. Automat. Contr.
2023
[Abs]
[doi]
[pdf]
A disturbance prediction based adaptive event-triggered model predictive control scheme is proposed for nonlinear systems in the presence of slowly varying disturbance. The optimal control problem in the model predictive control scheme is formulated by taking advantage of a proposed central path-based disturbance prediction approach, and the event-triggered mechanism is designed to be adaptive to the triggering interval. As a result, the proposed scheme improves the state prediction precision and hence reduces greatly the triggering frequency. Furthermore, for input-affine nonlinear systems, the disturbance separation and compensation techniques are developed to further enlarge the triggering interval. Theoretical analysis of the algorithm feasibility and closed-loop stability, as well as numerical evaluations of the effectiveness of the proposed schemes, are also given.
-
Event-Based Model Predictive Control for Nonlinear Systems with Dynamic Disturbance
Pengfei Li,
Tao Wang,
Yu Kang,
Kun Li,
and Yun-Bo Zhao
Automatica
2022
[Abs]
[doi]
[pdf]
In this paper, we investigate the event-based model predictive control (MPC) for constrained nonlinear systems with dynamic disturbance. An event-triggered disturbance prediction MPC (DPMPC) scheme and a self-triggered counterpart, which explicitly consider the disturbance dynamics, are proposed. For the event-triggered DPMPC scheme, the triggering condition relying on the state prediction error and the predicted disturbance sequence, updates at each time step based on the system states. For the self-triggered DPMPC scheme, the next triggering instant is determined by using the optimal state sequence and predicted disturbance sequence. In both event-based schemes, the optimal control problems are solved only at triggering instants, thus reducing the consumption of computational resources. The effectiveness of the two schemes is demonstrated by a simulation example.
-
A Novel Self-Triggered MPC Scheme for Constrained Input-Affine Nonlinear Systems
Pengfei Li,
Yu Kang,
Yun-Bo Zhao ,
and Tao Wang
IEEE Trans. Circuits Syst. II
2021
[Abs]
[doi]
[pdf]
This brief develops a novel self-triggered model predictive control algorithm based on time delay estimation for perturbed input-affine nonlinear systems. At each triggering instant, the algorithm determines simultaneously the predictive control sequence to feedforward compensate for the disturbance and the next triggering instant. As a consequence, the unnecessary samplings and transmissions are suppressed, and the frequency of solving the model predictive controller is reduced. The feasibility of the scheme as well as the associated stability are verified, with a numerical example illustrating the effectiveness of the proposed scheme.
-
Networked Dual-Mode Adaptive Horizon MPC for Constrained Nonlinear Systems
Pengfei Li,
Yu Kang,
Yun-Bo Zhao ,
and Tao Wang
IEEE Trans. Syst. Man Cybern, Syst.
2021
[Abs]
[doi]
[pdf]
This article investigates the predictive control scheme and related stability issue for a class of discrete-time perturbed nonlinear system with state and input constraints. First, we propose a novel control framework, i.e., networked dual-mode adaptive horizon model predictive control (MPC), which consists of a local controller, a remote controller that is subject to packet losses, and a judger coordinating the switchings between them. The optimization procedure of MPC with variable prediction horizon is implemented in the remote controller while a simple state-feedback control law is in the local one. Second, to establish the stability condition, we propose a new Lyapunov function. By specifying the relation between the Lyapunov function and the optimal MPC value function, the input-to-state practical stability is established. Finally, simulation results show the effectiveness of our proposed control scheme.
-
Robust Approximation-Based Event-Triggered MPC for Constrained Sampled-Data Systems
Tao Wang,
Yu Kang,
Pengfei Li,
Yun-Bo Zhao ,
and Peilong Yu
J Syst Sci Complex
2021
[Abs]
[doi]
[pdf]
In this paper, an approximation-based event-triggered model predictive control (AETMPC) strategy is proposed to implement event-triggered model predictive control for continuous-time constrained nonlinear systems under the digital platform. In our AETMPC strategy, both of the optimal control problem (OCP) and the triggering conditions are defined in discrete-time manner based on approximate discrete-time models, while the plant under control is continuous time. In doing so, sensing load is alleviated because the triggering condition does not need to be checked continuously, and the computation of the OCP is simpler since which is calculated in the discrete-time framework. Meanwhile, robust constraints are satisfied in continuous-time sense by taking inter-sampling behaviour into consideration, and a novel constraint tightening approach is presented accordingly. Furthermore, the feasibility the AETMPC strategy is analyzed and the associated stability of the overall system is established. Finally, this strategy is validated by a numerical example.
-
Robust Model Predictive Control for Constrained Networked Nonlinear Systems: An Approximation-Based Approach
Tao Wang,
Yu Kang,
Pengfei Li,
Yun-Bo Zhao ,
and Peilong Yu
Neurocomputing
2020
[doi]
[pdf]
Conference Articles
-
Equipment Health Assessment Based on AHP-CRITIC Dynamic Weight
Yunsheng Zhao,
Pengfei Li,
Tao Wang,
Yu Kang,
and Yun-Bo Zhao
In 2022 41st Chin. Control Conf. CCC
2022
[Abs]
[doi]
[pdf]
Prognostics Health and Management (PHM) has become a hot research problem with the improvement of different equipment. Besides, it is significant to assess the health status of equipment in PHM because an accurate health assessment can guide maintenance plans for engineers. To accurately reflect equipment health status by an index, an assessment method based on AHP-CRITIC dynamic weight is proposed in this paper. Analytic Hierarchy Process (AHP) is a subjective method used to evaluate the importance of different indicators. The criteria importance through inter-criteria correlation (CRITIC) method is used to calculate the contrast intensity of the same indicator and the conflict between indicators and obtain the objective weights. A set of more scientific weights is gained by combining the weights obtained from AHP and CRITIC, respectively. Moreover, to reflect each indicator’s real impact on overall health status, a dynamic weight adjustment mechanism is used. The case study of suction nozzles of a specific type of chip mounter shows that this method can reflect the health status accurately.
-
Self-Triggered Model Predictive Control for Perturbed Nonlinear Systems: An Iterative Implementation
Tao Wang,
Pengfei Li,
Yu Kang,
and Yun-Bo Zhao
In 2021 60th IEEE Conf. Decis. Control CDC
2021
[Abs]
[doi]
[pdf]
In this paper, a novel iterative self-triggered model predictive control strategy is proposed for continuous-time nonlinear systems with external disturbance. For this strategy, the triggering instants are determined by iteratively using the self-triggered mechanism. To be specific, the triggering mechanism, on the one hand, determines the next sampling instants of the sensor by a prespecified condition, and, on the other hand, decides whether or not to treat the current sampling instant as the triggering instant. Without continuous monitoring of the state, the sensing cost of the sensor can be alleviated. The utilization of the sampling states after the triggering instant leads to a larger triggering interval, and the computational load of the controller can thus be reduced. The effectiveness of the proposed strategy is validated by a numerical example.
-
Approximation-Based Self-Triggered Model Predictive Control for Perturbed Nonlinear Systems
Chang Xu,
Yu Kang,
Yun-Bo Zhao ,
Pengfei Li,
and Tao Wang
In 2021 China Autom. Congr. CAC
2021
[Abs]
[doi]
[pdf]
This paper proposes an approximation-based selftriggered model predictive control strategy for perturbed constrained nonlinear sampled-data systems. In our proposed strategy, the finite horizon optimal control problem (FHOCP) and the triggering condition are designed based on approximate discrete-time models. By implementing the strategy, the computation problem of the FHOCP becomes tractable since it is computed in a discrete-time framework. Meanwhile, the next triggering instant is pre-determined by the triggering condition, reducing the sensing cost and the computing frequency of the FHOCP. Furthermore, feasibility of the FHOCP and stability of the overall system are analyzed. Finally, a simulation example verifies the effectiveness of the strategy.
-
Event-Triggered Adaptive Horizon Model Predictive Control for Perturbed Nonlinear Systems
Pengfei Li,
Tao Wang,
Yu Kang,
and Yun-Bo Zhao
In 2020 59th IEEE Conf. Decis. Control CDC
2020
[Abs]
[doi]
[pdf]
This paper proposes a new event-triggered adaptive horizon model predictive control (MPC) for discrete-time nonlinear systems with additive disturbance. With the eventtriggered control scheme, the MPC is solved only at triggering instant and the event is triggered if the difference between the actual state and the predicted state exceeds the triggering threshold. The triggering threshold depends on the prediction horizon and becomes larger as the state approaches the terminal constraint set. Therefore, larger triggering intervals can then be obtained. Finally, a numerical example shows the effectiveness of the proposed scheme.
学位论文
Theses
-
面向分布式模型预测控制的事件驱动策略设计与分析
王涛
中国科学技术大学, 合肥
2023
[Abs]
[pdf]
分布式模型预测控制具有控制性能出色、多约束和多目标处理能力强、灵活 性和容错性高等优点,在智能电网、城市交通网络、工业控制等诸多领域得到了 广泛应用。在该类控制系统中,负责局部优化的节点的计算资源往往有限且节点 之间信息的传输容易受到网络资源的限制。这使得只在特定的时刻执行控制动 作的事件驱动策略成为分布式模型预测控制中的研究热点。 尽管已经取得一定的研究进展,但兼顾系统稳定性和算法可行性的低触发 策略仍有待研究,其核心原因是用于确保稳定性的 Lyapunov 函数递减原则和基 于不精确的状态预测误差保证可行性所必然带来的频繁触发问题。面向这一挑 战,本文从改变稳定性保证原则和提高预测误差精度的角度展开分布式模型预 测控制的事件驱动策略研究,具体工作包括: 1. 面向 Lyapunov 分析方法保守的触发策略设计,提出了自适应事件触发分 布式模型预测控制策略。定义了具有衰减预测时域的最优控制问题并基于 此设计了触发条件,降低了计算复杂度和触发频率,从而降低了计算和通 信负载。 2. 面向邻居信息非精确可知的触发策略设计,提出了复合事件触发分布式模 型预测控制策略,解决了在单一稳定性触发条件下频繁触发问题。设计了 与邻居系统估计信息无关的稳定性触发条件,其联合基于 Lyapunov 函数 的稳定性条件以并行触发的方式,降低了事件触发频率。 3. 面向预测模型不精确的触发策略设计,提出了基于扰动预测的自适应事件 触发分布式模型预测控制策略,提高了模型预测精度。设计了基于中心路 径的扰动预测方案和自适应触发阈值方案,降低了状态预测误差,提高了 触发阈值,显著降低了触发频率。4. 面向系统动态互联的触发策略设计,提出了滚动自触发分布式模型预测控 制策略,简化了优化问题设计,减小了状态预测误差估计的保守性。设计 了双模型最优控制问题,简化算法可行性分析的同时优化了控制性能。设 计了滚动自触发机制,增大了自触发的触发间隔,降低了计算和通信负载。 综上所述,本文对分布式模型预测控制的事件驱动策略设计和分析进行了 系统性的研究,创新地提出了对应的解决方案,推动了分布式模型预测控制的进 一步发展。
毕业去向
合肥工业大学, 讲师