研究背景
非完全信息动态博弈决策在复杂经济活动、人机对抗等领域有广泛用途,一直是博弈论研究的热点。在人工智能时代,数据量的大规模增长、机器智能的巨大飞跃和人机对抗等应用的飞速发展分别形成了“信息大数据”、 “算法高智能”和“对抗强动态”复杂环境,进一步导致了巨量数据不能直接利用的 “不可用”非完全信息形态、深度学习算法不可解释的“不可知”非完全信息形态和对抗欺骗状态下“不可信”非完全信息形态。这些全新的非完全信息形 态的出现使得非完全信息动态博弈决策理论面临着全新的挑战。应对这一挑战 一方面是对传统博弈理论在人工智能时代的重塑,另一方面也会带来全新的人工智能理论和技术的发展,在当前机遇窗口具有深远的意义。
研究目标
给出复杂环境下面向博弈决策的不同形态非完全信息的来源、种类和静态刻画, 提出信息大数据“不可用”、算法高智能“不可知”和对抗强动态“不可信”非完全信息下动态博弈决策的描述方法,并最终建立复杂环境下非完全信息博弈决策的智能基础模型。
主要研究内容
- 复杂环境下不同形态非完全信息的静态刻画
- 不同形态非完全信息下动态博弈决策的特征分析
- 非完全信息下博弈决策的智能基础模型构建
拟解决的重大科学问题或关键技术问题
- 理解复杂环境的“不确定性”:如何刻画复杂环境非完全信息并分析其对动态博弈模型的影响?
- 构建“不确定性”下的智能基础模型:如何将复杂环境 “不确定”特点融入到动态博弈框架下,构建新形式的智能基础模型?
- 智能基础模型的可用性:如何保证构建的智能基础模型满足所需的实时性要求,使得其实际可用?
基本研究框架
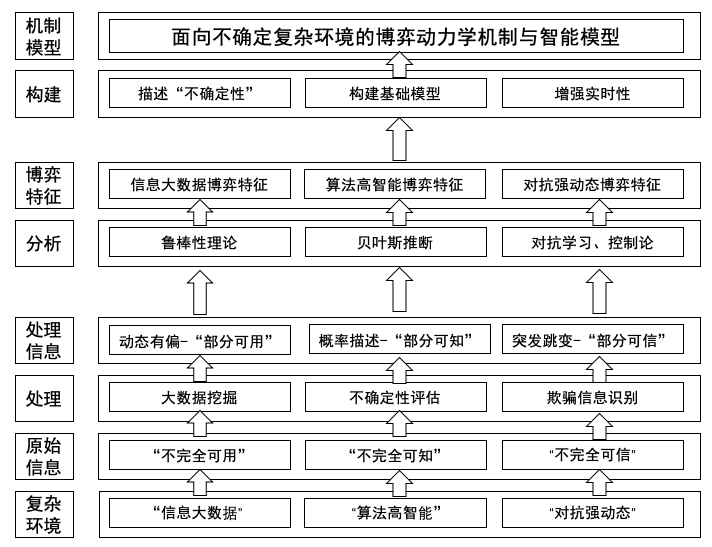
相关阅读
研究成果
Theses
-
基于人机信任的无人机竞速方法研究
陈少军
中国科学技术大学, 合肥
2024
[Abs]
[pdf]
无人机竞速技术在军事和民用领域展现出广泛应用潜力。在军事领域,无人 机竞速技术可以用于环境极速侦察、实施精准打击等;在民用领域,可以用于灾 难响应救援、工业管道检测等。因此,研究无人机的竞速方法具有重要意义。 竞速环境的复杂性给现有无人机竞速方法带来了挑战。一方面无人机在竞 速飞行中会遇到诸如湍流、阵风等不确定性因素,这种因素是突发、无征兆的, 难以通过概率模型进行建模;另一方面,在多机竞速飞行中,存在着诸如欺骗和 故意阻拦等高级博弈行为,这些行为因其复杂性而难以被精准定量描述。这些困 难会导致无人机自主决策出错,使得竞速效果显著下降。 人机混合决策在无人机竞速领域的应用备受关注,是解决上述挑战的潜在 方向。而人机混合有效决策高度依赖于良好的人机协作关系,否则会出现人类工 作负荷大、忽视机器错误决策等问题。考虑到人机信任起着协调人机协作的关 键作用,将人机信任融入人机混合决策为解决无人机竞速中的挑战提供了可能。 为此,论文提出了适用于无人机竞速场景的人机信任模型,基于该模型,设计了 单机场景和多机场景下的竞速策略。论文的主要研究工作分为以下三点:1)考虑到现有信任模型可解释性差、未能刻画竞速飞行高动态特点,提出 了机器性能驱动的人机信任模型,该模型能够促进人对机器的信任水平和机器 实际能力之间的匹配。首先给出了人机信任所具有的特征以及演化规律;其次, 引入机器当前表现作为机器性能的评估指标之一,构建机器性能驱动的人机信 任模型;最后设计了真实的人机交互实验用于验证所提模型。 (2)针对单机竞速场景中不确定性因素导致无人机竞速效果不佳的问题,提 出了基于人机信任的单机竞速共享控制策略,有效地改善了无人机竞速效果,同 时提升人类的容错率,降低人类工作量。首先设计用于检测人类失误的无人机轨 迹预测模块;其次构建基于人机信任的仲裁机制,实现人机权限的动态分配;最 后在实验平台上验证所提方法有效性。 (3)考虑到多机竞速场景中有着对于高级博弈行为的刻画和实时计算的需 求,提出了基于人机信任的多机竞速强化学习策略,该方法能够实时给出决策指 令,同时提升了机器对于博弈的理解和竞速效果。首先将人机信任作为训练奖励 融入到奖励塑造函数中;其次设计对手轨迹预测网络,增加机器对于对手的策略 理解;最后设计实验验证所提方法有效性。
-
基于训练和执行双阶段联合设计的人机智能决策方法研究
李明
中国科学技术大学, 合肥
2023
[Abs]
[pdf]
在人机混合智能系统中,人工智能赋能的机器智能和人类智能相互融合,在 特定场景下可以超越单独人类或者机器的决策性能,成为当前的研究热点。但 是,与传统的人机系统和人工智能算法不同,人机混合智能系统的决策效果不仅 受到训练阶段人工智能算法性能的影响,比如算法的泛化性和鲁棒性,而且也会 受到执行阶段人类和机器决策混合方法的影响,比如人类和机器控制权的分配。 如何从整体上优化人机混合智能系统的决策性能,是当下重要的研究课题。 本文面向深度强化学习算法驱动的人机混合智能决策系统的序贯决策问题, 同时从算法的训练端和执行端出发,通过引入人类智能的方式提高系统决策的 鲁棒性和安全性,最终提高人机混合智能系统的决策性能。本文工作主要包含以 下三个方面: (1) 针对强化学习算法驱动的人机共享控制系统的序贯决策问题,在训练阶 段提出了基于人类策略限制下人在环上强化学习算法,避免机器做出危险的行 为,同时提高了算法的采样效率;在执行阶段提出了包含人类决策评估的仲裁机 制,舍弃了人类错误的决策,提高了系统的整体性能。实验结果表明,此方法成 功提高了算法训练的采样效率和系统执行任务的成功率。 (2) 针对多机竞速场景下强化学习算法驱动的人机介入控制系统的序贯决策 问题,在训练阶段引入了包含人类反馈奖励的奖励函数组,以引导机器理解竞速 规则,减少了执行阶段人类的介入次数;在执行阶段引入了人类的两级介入机 制,避免违背规则或者容易造成事故的行为出现,同时降低了人类介入时的操作 负担。实验结果表明,此方法缩短了无人机的单圈耗时,提高了系统决策的安全 裕度,并且减轻了人类的介入负担。 (3) 针对上述人机混合序贯决策方法,本文以旋翼无人机为背景,搭建了从 仿真到现实的人机实验平台,提出了算法部署到真实物理场景的整体流程和框 架,并针对提出的多机竞速场景下强化学习算法驱动的人机介入控制方法,进行 了现实场景下的算法验证。
-
基于博弈模型的无人机机动决策方法研究
殷书慧
中国科学技术大学, 合肥
2023
[Abs]
[pdf]
无人机作为未来战场的核心力量对于夺取制空权起到至关重要的作用,其 自主机动决策能力是发挥作战效能的关键所在。现有的空战决策方法诸如微分 对策、专家系统等虽取得一定成果,但仍存在着搜索决策结果耗时长、适应性差 等局限性。因此,如何在高动态、强竞争性的无人机对抗环境下进行快速准确的 机动决策是本论文主要研究的问题。 本文以近距对抗为背景,以博弈理论为基础,以智能算法为工具,围绕基于 博弈模型的无人机机动决策方法展开研究,具体研究工作如下: (1)基于 F-16 机型无人机进行控制参数设计,并在此基础上对基本操纵动 作库进行丰富和改进,设计了无人机的机动空间,构建了无人机的机动策略集。 仿真实验分别对所设计的控制参数和机动空间进行测试,结果都满足设计需求。 (2)针对基本群智算法搜索决策结果计算效率低且容易陷入局部最优值的 问题,提出了一种改进粒子群算法求解最优机动策略。首先,建立了无人机一对 一动态博弈模型。然后,将博弈混合策略纳什均衡难于求解的问题转化为最优化 问题进行搜索寻优,提出了一种改进的群体智能优化算法,通过粒子浓度的概率 选择来控制种群多样性,以降低在优化收敛阶段陷入局部最优值的可能性。最后 将其应用到无人机对抗机动决策中,设计了单机对抗仿真实验对比改进后算法 的性能,结果表明改进粒子群算法提升了全局搜索效率和寻优精度,提高了无人 机对抗机动决策中求解最优机动策略的计算效率和准确度。(3)针对传统强化学习算法在处理高维状态输入时存在的维数爆炸问题,以 及倾向于单方面最优化自身策略而不考虑对手策略影响的问题,提出了一种改 进 DQN 算法生成有效对抗决策。首先,建立了无人机一对一场景下的二人零和 马尔可夫博弈模型,并据此设计了一对一场景的基本状态空间、动作空间和奖 励函数。然后,针对高维状态输入,引入深度神经网络拟合状态动作值函数,通 过设置经验回放技巧并利用损失函数更新网络参数,提高了算法的收敛性和稳 定性。其次,针对单方优化问题,引入博弈决策的极大极小均衡来生成针对性机 动策略。最后,设计了单机对抗仿真实验对比改进后算法的性能,结果表明改进 DQN 算法可以通过自学习的方式在强竞争环境下生成更准确、更有效针对对手 的机动决策,满足对抗实时性的同时具有更高的决策水平。
-
基于人类决策有效性的人机混合决策方法研究
游诗艺
中国科学技术大学, 合肥
2022
[Abs]
[pdf]
随着人工智能技术的发展,机器的自主能力不断地提高,智能机器在各行各 业的应用和发展日益深入。在此进程中,不可避免地会遇到智能机器无法应对 实际任务的复杂性和不可预测性的情况,许多系统在未来仍将需要人类在监督、 目标设定、应急响应等方面与机器进行持续、密切的交互,研究此种场景下如何 混合人类决策和机器决策以达到更好的决策效果也因此尤为重要和有意义。 在人机混合决策中,人类决策是否有效,即人的决策是否促进任务的完成并 有效地反映人类的真实意图,从两方面影响着最终的决策性能。一方面在于一方 决策失效将导致混合性能的下降;另一方面在于智能机器常常无法直接得知人 的意图,而需先根据人类决策推测意图,再做出决策辅助人完成该意图,人类决 策的失效可能导致意图推理的失效,进而导致人机混合决策方法的失效和任务 失败。因此本文以人机混合决策方法为研究对象,基于人类决策的有效性,从人 类决策全时有效和人类决策非全时有效两个方面展开研究,提出基于强化学习 的人机混合决策方法来改善决策性能。本文的研究工作主要包括以下两个方面: (1)针对人类决策全时有效的情况,提出一种基于最小干预原则的人机混合 决策方法,在优化整体系统性能的基础上,进一步考虑人对于人机系统满意度的 相关指标。通过将最小干预原则引入人机混合决策,设置人机决策融合的自适应 阈值,该方法能够以最小程度的干预为人类提供最大程度的帮助,并能在实时变 化的环境中保持最优,同时提升和改善系统性能和人类满意度两类指标,为后续 的优化设计方案提供基础性方法。 (2)针对人类决策非全时有效的情况,提出一种基于人类决策有效性评估机 制的人机混合决策方法,以避免人的无效决策损害系统性能。通过利用强化学习 算法判断人类决策的有效性,识别人的意图是否改变,该方法能够在人类决策无 效时由机器单独完成任务,使得系统在人类决策非全时有效的情况下,仍能完成 正确的任务目标,有效提升了人机混合决策质量和系统性能。
-
基于机器视觉的驾驶员注意力检测系统设计
唐敏
浙江工业大学, 杭州
2021
[Abs]
[pdf]
驾驶员注意力不集中是指驾驶员的注意力被一些分心驾驶行为所分散从而 不集中。车辆驾驶过程中的注意力分散行为将会使得驾驶员的反应过程被拉长, 进而使得驾驶员对车辆能否安全行驶的判断能力和对突发情况的反应能力降低, 极易引起交通安全事故。近年来,驾驶员注意力检测已经越来越多地受到大家 的关注。 本文针对驾驶员注意力检测场景,设计了基于机器视觉的驾驶员注意力检 测系统,进行了驾驶员注意力检测系统的软硬件设计,设计的基于多粒度特征 和中间层特征的 MGMN 算法在现有的公开的分心驾驶数据集中取得了更高的精 确度,设计的硬件系统成本较低并且在真实场景实验中也有着较高的实用性。 本文的主要工作有以下两个方面: (1) 设计了基于多粒度特征和中间层特征的驾驶员注意力检测算法,该算法 具有精度高、特征提取丰富且准确的特点。考虑到驾驶员在驾驶时头部和手部 都处于图像的相对固定的位置,并且头部一般处于图片的上方,手部一般处于 图片的中下方。如果可以针对驾驶图像的每一部分进行学习,提取每一部分特 有的特征,本文在 ResNet50 模型中加入了多粒度特征提取部分,该部分有全局 分支、二分支和三分支三部分,共同提取驾驶图像的全局和局部特征。同时又 抽取了驾驶图像的中间层特征,中间层包含了丰富的图像特征,最后将多粒度 特征和中间层特征一起输入到 Softmax 损失函数中,设计出了 MGMN 算法。 MGMN 算法无论是在现有的分心驾驶检测公开数据集上还是在自建数据集上都 有着更高的精确度和较短的运行时间。 (2)设计了“树莓派 +远程服务器”的驾驶员注意力检测系统。 采用“树莓 派+远程服务器”的结构,通过局域网将树莓派与远程服务器相连接,具有较高 的信息传输速率,树莓派与服务器之间采用 Socket 通信方式进行通信,做到终 端与服务器之间的信息的准确传输。同时采用成本低、体积小、可靠性高的树 莓派作为驾驶图像实时采集终端,采用配置高、运行效率高的服务器作为状态 判别的设备,降低了设计成本,实现了对驾驶员注意力状态实时的高精度检测。
-
面向人机序贯决策的混合智能方法研究
张倩倩
中国科学技术大学, 合肥
2021
[Abs]
[pdf]
随着人工智能技术的发展,机器智能得到不断的提高,随之而来的则是机 器智能得以在各行各业应用发展。在此进程中,不可避免的会遇到机器自主性 不足以解决本身该由人类解决或者人类必须参与决策的情况,考虑此种场景下 人类智能和机器智能共同作用的决策问题则显得尤为重要和有意义。更具体地, 序贯决策问题作为一类具有时序性和多阶段性的动态决策问题,其发展与当下 人工智能时代下的工程应用、生产生活等领域息息相关。人的作用体现在序贯决 策问题的两方面,一则,人本身属于序贯决策问题模型中的一部分,即该类问题 是离不开人的如微创外科手术等;二则,人的相关信息不体现在序贯决策问题模 型中,而是因人独特的认知能力使得其可以出现在问题的求解办法中,达到改善 问题求解的目的如人对机器搜救系统的引导等,我们将上述两种场景统称为 “人 机序贯决策问题”。 针对人机序贯决策问题,由于人类智能和机器智能本质上的区别,数学表达 上的巨大差异,使得人和机器共同作用于问题求解时,不可避免的因为协调原因 造成决策质量不高甚至决策失误的现象。然而直接应用传统人机系统的控制算 法不能有效处理这些问题,从而引起机器代理失效,人力浪费,甚至还会造成决 策系统性能恶化甚至崩溃。因此,亟需设计有效的人机混合智能算法来解决这些 问题。本文以人机序贯决策问题为研究对象,围绕人机混合智能控制中的决策权 限划分、介入控制触发切换时机和共享控制混合人机决策动作程度三个问题展 开研究,旨在提出有效的人机混合智能算法来改善提升人机序贯决策问题的求 解。本文的研究工作主要包括以下几个方面: 1. 提出了基于强化学习方法的人机混合智能控制框架。通过将机器代理的决 策和人类的决策以可信性和安全性为评价指标进行仲裁选择,以确定更优 的待执行决策动作。同时考虑了基于模型的强化学习子系统和基于无模型 的强化学习子系统,为适应广泛的序贯决策应用场景提供了更多可能。 2. 针对人机序贯决策中的介入控制问题,提出了自主性及自主性边界的概念, 通过将自主性边界的求解形式化为与任务目标相关的常规优化问题进行讨 论判定,优化介入控制的控制方案和算法,实现人机序贯决策中人介入机 器场景和机器介入人场景下的决策性能提升。 3. 针对人机序贯决策中的共享控制问题,提出了基于自主性边界的混合参数 优化设计方案,通过自适应调节混合参数大小直接影响最终待执行动作的 生成。考虑了人机动作的融合程度,使得最优解在人的动作空间和机器的 动作空间所共同张成的扩展空间中出现,为决策质量的提升提供了扩展空间。 4. 针对介入控制和共享控制中所估计的自主性边界值可能存在单值估计不准 确的问题,提出了基于贝叶斯神经网络的不确定性估计办法,获得自主性 边界的概率分布信息并用于决策动作生成,利用自主性边界的不确定性优 化设计人机混合智能算法,既使得决策动作的优化存在更多选择,也更加 符合人们对决策边界的模糊性思考。 综上所述,本文面向人机序贯决策对混合智能算法所面临的问题进行了系 统性的研究,创新性地提出了对应的解决方案,推动了人机序贯决策求解和混合 智能算法的进一步发展。
-
无界 DoS 攻击下网络化控制系统的防护设计
朱巧慧
浙江工业大学, 杭州
2021
[Abs]
[pdf]
由于开放、共享通信网络易于遭受攻击,安全问题已成为网络化控制系统 近些年关注的焦点之一,其中拒绝服务攻击(DoS)是最常见和易于实现的攻 击形式,得到了广泛的关注。现有研究大多假设 DoS 攻击在频率、持续时间或 其他指标上是有限的,这对现实通信系统是合理的;但对控制系统而言,只要 攻击强度超出了所能承受的界限,控制系统就会因为长时间开环而失稳,这意 味着对遭受 DoS 攻击的网络化控制系统而言,带有无界假设的 DoS 攻击具有重 要的现实意义,需要针对该类系统展开建模、分析和设计等研究。 在基于国内外研究的基础上,针对带有无界 DoS 攻击的网络化控制系统给 出了相应的解决方案,具体工作包括: (1)针对无界 DoS 攻击下的网络化控制系统,提出了一种多路径切换防护 策略。首先设计 DoS 攻击检测模块对当前路径进行攻击检测,然后设计多路径 切换模块进行路径的切换。进一步将全局均方渐近稳定的概念引入到闭环控制 系统中,得到了闭环系统的全局均方渐近稳定条件以及给出了闭环系统控制器 增益的设计方法。仿真结果证明了多路径切换防护策略有效降低了无界 DoS 攻 击对控制系统的影响。 (2)针对无界 DoS 攻击下受扰网络化控制系统,基于多路径切换防护策略, 得到了闭环系统的均方一致最终有界的稳定性条件,并进一步给出了控制器增 益设计方法。仿真结果证明了抗扰控制器设计方法具有更强的鲁棒性。
Journal Articles
-
A Novel Prescribed-Time Control Approach of State-Constrained High-Order Nonlinear Systems
Yangang Yao,
Yu Kang,
Yunbo Zhao,
Pengfei Li,
and Jieqing Tan
IEEE Trans. Syst. Man Cybern, Syst.
2024
[Abs]
[doi]
[pdf]
A novel practical prescribed-time control (PPTC) approach for high-order nonlinear systems (HONSs) subject to state constraints is studied in this article. Different from the existing methods which always require the constraint boundaries to be continuous functions, the state constraints considered in this article are discontinuous (i.e., the state constraints occur only in some time periods and not in others), which can be found in many practical systems. By designing a novel stretch modelbased nonlinear mapping function (NMF), the state constraints are dealt with directly, and the limitations that the virtual control function depends upon the feasibility condition (FC) and the tracking error depends upon the constraint boundaries in the conventional schemes are removed. Meanwhile, the proposed method is a unified one, which is also effective for HONSs with conventional continuous state constraints/ deferred state constraints/ funnel constraints or constraints-free without altering the control structure. Furthermore, by designing a newly timevarying scaling transformation function (STF), a more relaxed criterion for practical prescribed-time stable (PPTS) is given, based on which a newly PPTC algorithm is designed. The result shows that the proposed algorithm can preset the upper bound of the settling time, which does not depend upon the initial state of the system and control parameters, the limitations of singularity problem and excessive initial control input in existing methods are removed. Simulation examples verify the algorithm developed.
-
基于动态信道切换的无线网络化控制系统的资源调度策略
郝小梅,
and 赵云波
高技术通讯
2023
[Abs]
[pdf]
本文针对通信网络中存在竞争和非竞争信道的无线网络化控制系统,提出了一种基于估计 器的信道选择策略,在保证控制系统稳定性的同时尽可能地节约了宝贵的非竞争信道资源。在无 线网络化控制系统中,控制信号通过竞争信道传输时可能发生数据包丢失,导致执行器无法收到 实时的控制信号。而传感器端未知控制信号的实际传输情况,因而也无法得知每个时刻执行器所 使用的控制信号。针对这种情况,本文首先设计了估计器来估计执行器端上一时刻实际使用的控 制信号,再通过信道选择策略来约束执行器端使用控制信号的误差。然后,在所提信道选择策略 下设计控制器来保证控制系统稳定。最后,通过数值仿真验证了所提算法的有效性。
-
Compound Event-Triggered Distributed MPC for Coupled Nonlinear Systems
Yu Kang,
Tao Wang,
Pengfei Li,
Zhenyi Xu,
and Yun-Bo Zhao
IEEE Trans. Cybern.
2023
[Abs]
[doi]
[pdf]
This paper investigates the event-triggered distributed model predictive control (DMPC) for perturbed coupled nonlinear systems subject to state and control input constraints. A novel compound event-triggered DMPC strategy, including a compound triggering condition and a new constraint tightening approach, is developed. In this event-triggered strategy, two stability-related conditions are checked in a parallel manner, which relaxes the requirement of the decrease of the Lyapunov function. As a result, the number of triggering instants can be reduced significantly. Furthermore, the proposed constraint tightening approach solves the problem of the state constraint satisfaction, which is quite challenging due to the external disturbances and the mutual influences caused by dynamical coupling. Simulations are conducted at last to validate the effectiveness of the proposed algorithm.
-
Robust Nonsingular Fixed Time Terminal Sliding Mode Control for Atmospheric Pollution Detection Lidar Scanning Mechanism
Yu Kang,
Yuxiao Yang,
Cai Chen,
Wenjun Lü,
and Yunbo Zhao
J Syst Sci Complex
2023
[Abs]
[doi]
[pdf]
A robust nonsingular fixed time terminal sliding mode control scheme with a time delay disturbance observer is proposed for atmospheric pollution detection lidar scanning mechanism (APDL-SM) system. Distinguished from the conventional terminal sliding mode control methods, the authors design a novel fixed-time terminal sliding surface, the convergence time of sliding mode phase of which has a constant upper bound that is designable by adjusting only one parameter. Moreover, in order to overcome the problem of unknown upper bound of lumped uncertainty including model uncertainty, friction effect and external disturbances from the port environment, the authors propose a time delay disturbance observer to provide an estimation for the system lumped uncertainty. By using the Lyapunov synthesis, the explicit analysis of the convergence time upper bound are performed. Finally, simulation studies are conducted on the APDL-SM system to show the fast convergence rate and strong robustness of the proposed control scheme.
-
Disturbance Prediction-Based Adaptive Event-Triggered Model Predictive Control for Perturbed Nonlinear Systems
Pengfei Li,
Yu Kang,
Tao Wang,
and Yun-Bo Zhao
IEEE Trans. Automat. Contr.
2023
[Abs]
[doi]
[pdf]
A disturbance prediction based adaptive event-triggered model predictive control scheme is proposed for nonlinear systems in the presence of slowly varying disturbance. The optimal control problem in the model predictive control scheme is formulated by taking advantage of a proposed central path-based disturbance prediction approach, and the event-triggered mechanism is designed to be adaptive to the triggering interval. As a result, the proposed scheme improves the state prediction precision and hence reduces greatly the triggering frequency. Furthermore, for input-affine nonlinear systems, the disturbance separation and compensation techniques are developed to further enlarge the triggering interval. Theoretical analysis of the algorithm feasibility and closed-loop stability, as well as numerical evaluations of the effectiveness of the proposed schemes, are also given.
-
Leader-Following Cluster Consensus of Multiagent Systems With Measurement Noise and Weighted Cooperative–Competitive Networks
Cui-Qin Ma,
Tian-Ya Liu,
Yu Kang,
and Yun-Bo Zhao
IEEE Trans. Syst. Man Cybern, Syst.
2023
[Abs]
[doi]
[pdf]
Leader-following cluster consensus is investigated for multi-agent systems with weighted cooperative-competitive networks and measurement noise. A stochastic approximation protocol is proposed for interactively balanced and sub-balanced networks, and pinning control is introduced to deal with the divergence phenomenon in interactively unbalanced networks. With these protocols, sufficient conditions for reaching strong mean square leader-following cluster consensus are established for all the three types of networks, which are also extended to the cases without measurement noise. Numerical examples illustrate the effectiveness of the proposed protocols and theoretical analysis.
-
基于轨迹预测与改进人工势场法的机械臂动态避障规划方法
吴芳,
and 赵云波
高技术通讯
2023
[Abs]
[pdf]
在人机共存环境中,人可能会成为机器人执行任务过程中的动态障碍物,因此, 在机器人的运动过程中需要动态地避障规划,从而避免机器人危害到用户安全。 人工势 场法是常用的动态路径规划算法,具有实现简单、计算实时性高等优点。 传统的人工势场 法根据虚拟的引力场和斥力场得到合力,从而引导机器人的运动,但是当引力和斥力等大 反向时,存在局部极小值问题。 针对该问题,本文提出了基于平面位置采样的改进人工势 场法。 每次计算得到合力向量后,以该向量的指向为中心,在垂直于地面、包含合力向量 的平面上以特定的角度间隔分别逆时针和顺时针方向采样 90 °范围内的运动方向,然后 分别计算引力值和斥力值,最后根据引力值和斥力值的加权和最小确定机器人的最佳运 动方向。 为了应对用户运动导致机器人运动路径突变的情况,本文依据用户手臂运动和 头部转动的关联关系,通过检测用户的头部姿态,并利用手臂当前的运动信息预测手臂接 下来的运动位置。 最后设计了基于轨迹预测与改进人工势场法的机械臂动态避障规划方 法,实验结果表明,该方法可以有效地进行动态避障,并且规划的路径更加平滑、长度更短。
-
基于丢包率估计的无线网络化控制系统的逼近控制策略
吴芳,
梁启鹏,
叶睿卿,
and 赵云波
高技术通讯
2023
[Abs]
[pdf]
本文针对丢包为分段伯努利过程的无线网络化控制系统进行了控制器设计和稳定性分析。丢包满足分段伯努利过程是指未知丢包率将在未知时刻突变到另一个未知概率上并保持一段时间。针对这一丢包特点,本文提出了基于丢包率估计的逼近控制策略以保证系统稳定性。首先设计了丢包率估计器和逼近控制器,使系统在线估计丢包率,并利用丢包率估计得到控制量。然后为平衡系统性能和网络信道资源设计了信道调度机制。最后设计了丢包率突变检测器使系统自适应丢包率突变。在此基础上得到了保证闭环系统均方最终一致有界的充分条件和控制增益计算方法。数值仿真验证了控制策略的有效性。
-
基于优先级预测器的无线网络化控制系统的动态传输策略
闫文晓,
and 赵云波
高技术通讯
2023
[Abs]
[doi]
[pdf]
文针对无线通信网络中存在丢包的多包传输无线网络化控制系统,提出了一种基于预测 器的动态传输策略,在几乎不增加信道资源占用的情况下显著提升系统稳定性。在多包传输的无 线网络化控制系统中,由于通信资源的限制,传感器到控制器间的数据传输中出现丢包问题,影 响控制系统性能。针对这个问题,本文首先设计了优先级预测器来预测下一时刻每个传感器数据 对系统稳定性的影响,帮助系统决策每个传感器的发送优先级,再通过传输调节器对不同优先级 传感器补偿相应的随机退避时间上限,进而让优先级高的传感器在随机退避的方式下优先传输, 然后在此策略下设计控制器使系统稳定。最后通过数值仿真验证了本文策略的有效性。
-
面向人机序贯决策实现共享控制下的仲裁优化
张倩倩,
赵云波,
吕文君,
and 陈谋
中国科学:信息科学
2023
[Abs]
[doi]
[pdf]
共享控制存在于众多由人类智能和机器智能共同参与的序贯决策场景. 由于人的决策范围和 智能机器的决策范围尚未予以明确划分, 需要加以实时仲裁从而达到人机共存并且共享决策权限. 为 此本文提出了一种仲裁优化方法, 该方法的独特之处在于引入自主性边界概念, 优化了共享控制中人 机决策动作的仲裁机制. 本文为自主性边界的计算和更新维护提供了思路, 能够基于贝叶斯规则的意 图推理分析人机共享系统可能要实现的目标, 从而确定仲裁参数. 此外, 本文还分析了自主性边界的 不确定性以促进边界信息对共享控制中决策质量的优化效果. 实验结果表明, 所提出的方法在累积奖 励、成功率、撞击率方面表现出色, 这些说明了本文提出的共享控制中的仲裁优化方法在求解人机序 贯决策问题时的有效性和价值.
-
非完全信息下人机合作对抗博弈专题编者按
康宇,
段海滨,
and 赵云波
中国科学:信息科学
2022
[doi]
[pdf]
-
Integrated Channel-Aware Scheduling and Packet-Based Predictive Control for Wireless Cloud Control Systems
Pengfei Li,
Yun-Bo Zhao ,
and Yu Kang
IEEE Trans. Cybern.
2022
[Abs]
[doi]
[pdf]
The scheduling and control of wireless cloud control systems involving multiple independent control systems and a centralized cloud computing platform are investigated. For such systems, the scheduling of the data transmission as well as some particular design of the controller can be equally important. From this observation, we propose a dual channel-aware scheduling strategy under the packet-based model predictive control framework, which integrates a decentralized channel-aware access strategy for each sensor, a centralized access strategy for the controllers, and a packet-based predictive controller to stabilize each control system. First, the decentralized scheduling strategy for each sensor is set in a noncooperative game framework and is then designed with asymptotical convergence. Then, the central scheduler for the controllers takes advantage of a prioritized threshold strategy, which outperforms a random one neglecting the information of the channel gains. Finally, we prove the stability for each system by constructing a new Lyapunov function, and further reveal the dependence of the control system stability on the prediction horizon and successful access probabilities of each sensor and controller. These theoretical results are successfully verified by numerical simulation.
-
Event-Based Model Predictive Control for Nonlinear Systems with Dynamic Disturbance
Pengfei Li,
Tao Wang,
Yu Kang,
Kun Li,
and Yun-Bo Zhao
Automatica
2022
[Abs]
[doi]
[pdf]
In this paper, we investigate the event-based model predictive control (MPC) for constrained nonlinear systems with dynamic disturbance. An event-triggered disturbance prediction MPC (DPMPC) scheme and a self-triggered counterpart, which explicitly consider the disturbance dynamics, are proposed. For the event-triggered DPMPC scheme, the triggering condition relying on the state prediction error and the predicted disturbance sequence, updates at each time step based on the system states. For the self-triggered DPMPC scheme, the next triggering instant is determined by using the optimal state sequence and predicted disturbance sequence. In both event-based schemes, the optimal control problems are solved only at triggering instants, thus reducing the consumption of computational resources. The effectiveness of the two schemes is demonstrated by a simulation example.
-
Cluster Consensus for Coupled Harmonic Oscillators Under a Weighted Cooperative-Competitive Network
Cui-Qin Ma,
Tian-Ya Liu,
and Yun-Bo Zhao
International Journal of Control
2022
[Abs]
[doi]
[pdf]
Cluster consensus is investigated for multiple coupled harmonic oscillators under a weighted cooperativecompetitive network. Consensus protocols for three categories of communication networks are constructed by employing a weighted gain, and sufficient conditions for guaranteeing cluster consensus are obtained. It is found that under the proposed protocols, the states of all oscillators can be guaranteed to reach periodic orbits that are the same in frequency no matter which cluster the oscillators belong to. In particular, cluster partitions here are not given a prior, but are determined by the communication topology among oscillators. Numerical examples are given to validate the effectiveness of theoretical results.
-
非全时有效人类决策下的人机共享自主方法
游诗艺,
康宇,
赵云波,
and 张倩倩
中国科学:信息科学
2022
[Abs]
[doi]
[pdf]
在人机共享自主中, 人和智能机器以互补的能力共同完成实时控制任务, 以实现双方单独控制 无法达到的性能. 现有的许多人机共享自主方法倾向于假设人的决策始终“有效”, 即这些决策促进了 任务的完成, 且有效地反映了人类的真实意图. 然而, 在现实中, 由于疲劳、分心等多种原因, 人的决 策会在一定程度上“无效”, 不满足这些方法的基本假设, 导致方法失效, 进而导致任务失败. 在本文 中, 我们提出了一种新的基于深度强化学习的人机共享自主方法, 使系统能够在人类决策长期无效的情况下完成正确的目标. 具体来说, 我们使用深度强化学习训练从系统状态和人类决策到决策价值的 端到端映射, 以显式判断人类决策是否无效. 如果无效, 机器将接管系统以获得更好的性能. 我们将该 方法应用于实时控制任务中, 结果表明该方法能够及时、准确地判断人类决策的有效性, 分配相应的 控制权限, 并最终提高了系统性能.
-
Traded Control of Human–Machine Systems for Sequential Decision-Making Based on Reinforcement Learning
Qianqian Zhang,
Yu Kang,
Yun-Bo Zhao ,
Pengfei Li,
and Shiyi You
IEEE Trans. Artif. Intell.
2022
[Abs]
[doi]
[pdf]
Sequential decision-making (SDM) is a common type of decision-making problem with sequential and multistage characteristics. Among them, the learning and updating of policy are the main challenges in solving SDM problems. Unlike previous machine autonomy driven by artificial intelligence alone, we improve the control performance of SDM tasks by combining human intelligence and machine intelligence. Specifically, this article presents a paradigm of a human–machine traded control systems based on reinforcement learning methods to optimize the solution process of sequential decision problems. By designing the idea of autonomous boundary and credibility assessment, we enable humans and machines at the decision-making level of the systems to collaborate more effectively. And the arbitration in the humanmachine traded control systems introduces the Bayesian neural network and the dropout mechanism to consider the uncertainty and security constraints. Finally, experiments involving machine traded control, human traded control were implemented. The preliminary experimental results of this article show that our traded control method improves decision-making performance and verifies the effectiveness for SDM problems.
-
利用人的分歧介入增强珍珠自动分拣可靠性研究
花婷婷,
王岭人,
and 赵云波
计算机测量与控制
2021
[Abs]
[pdf]
面向珍珠自动分拣应用场景,研究提出了一种通过人的分歧介入提升分拣可靠性的方法。该方法引入两个独立 AI 系统用于珍珠分拣的预处理,然后通过二者之间的分歧引入人的介入干预,在较少的人力成本下达到了对机器算法可 靠性的提升。定义了包括分歧准确指数和额外成本指数在内的性能评价指标,在公开的珍珠数据集上,研究提出的方法以 4.1%的额外人工成本提升了近 4%的珍珠分拣精度,验证了方法的有效性。
-
A Novel Self-Triggered MPC Scheme for Constrained Input-Affine Nonlinear Systems
Pengfei Li,
Yu Kang,
Yun-Bo Zhao ,
and Tao Wang
IEEE Trans. Circuits Syst. II
2021
[Abs]
[doi]
[pdf]
This brief develops a novel self-triggered model predictive control algorithm based on time delay estimation for perturbed input-affine nonlinear systems. At each triggering instant, the algorithm determines simultaneously the predictive control sequence to feedforward compensate for the disturbance and the next triggering instant. As a consequence, the unnecessary samplings and transmissions are suppressed, and the frequency of solving the model predictive controller is reduced. The feasibility of the scheme as well as the associated stability are verified, with a numerical example illustrating the effectiveness of the proposed scheme.
-
A Novel Inertial-Visual Heading Determination System for Wheeled Mobile Robots
Wenjun Lv,
Yu Kang,
Yun-Bo Zhao ,
Yuping Wu,
and Wei Xing Zheng
IEEE Trans. Contr. Syst. Technol.
2021
[Abs]
[doi]
[pdf]
Finding an alternative way to replace the magnetic compass to determine the robot heading angle indoor is always a challenge in the robotics society. This paper proposes a structurally simple yet efficient non-magnetic heading determination system, which can be used in the planar indoor environment with abundant ferro- and electro-magnetic interferences, by the combination of gyroscope and vision. The gyroscope is utilized to perceive the yaw rate, while a downward-looking camera is used to capture the pre-laid auxiliary strips to acquire the absolute angle of the robot heading. Due to the existence of pseudo measurement, varying noise statistical characteristics, and asynchronization between state propagation and measurement, the existing Kalman filters cannot be applied to fuse the gyroscopic and visual data. Therefore, a novel fusion algorithm named pseudo-measurement-resistant adaptive asynchronous Kalman filter is proposed, which is experimentally verified to be efficient in the environment with various interferences.
-
Robust Approximation-Based Event-Triggered MPC for Constrained Sampled-Data Systems
Tao Wang,
Yu Kang,
Pengfei Li,
Yun-Bo Zhao ,
and Peilong Yu
J Syst Sci Complex
2021
[Abs]
[doi]
[pdf]
In this paper, an approximation-based event-triggered model predictive control (AETMPC) strategy is proposed to implement event-triggered model predictive control for continuous-time constrained nonlinear systems under the digital platform. In our AETMPC strategy, both of the optimal control problem (OCP) and the triggering conditions are defined in discrete-time manner based on approximate discrete-time models, while the plant under control is continuous time. In doing so, sensing load is alleviated because the triggering condition does not need to be checked continuously, and the computation of the OCP is simpler since which is calculated in the discrete-time framework. Meanwhile, robust constraints are satisfied in continuous-time sense by taking inter-sampling behaviour into consideration, and a novel constraint tightening approach is presented accordingly. Furthermore, the feasibility the AETMPC strategy is analyzed and the associated stability of the overall system is established. Finally, this strategy is validated by a numerical example.
-
Multi-Path Switching Protection for Networked Control Systems Under Unbounded DoS Attacks
Qiaohui Zhu,
Qipeng Liang,
Yu Kang,
and Yun-Bo Zhao
Journal of University of Science and Technology of China
2021
[Abs]
[doi]
[pdf]
The strategy design and closed-loop stability of networked control systems under unbounded denial of service (DoS) attacks are investigated. A multi-path switching protection strategy is firstly designed by noticing the usually available multiple paths in data communication networks. The strategy consists of a DoS attack detection module at the actuator side to identify DoS attacks from normal data packet dropouts, and a multi-path switching module at the sensor side to effectively switch the data transmission path when necessary. Then, the sufficient conditions for the closed-loop system being global mean square asymptotic stability are given, with a corresponding controller gain design method. Numerical examples illustrate the effectiveness of the proposed approach.
-
Robust Model Predictive Control for Constrained Networked Nonlinear Systems: An Approximation-Based Approach
Tao Wang,
Yu Kang,
Pengfei Li,
Yun-Bo Zhao ,
and Peilong Yu
Neurocomputing
2020
[Abs]
[doi]
[pdf]
In this paper, a robust approximation-based model predictive control (RAMPC) scheme for the constrained networked control systems (NCSs) subject to external disturbances is proposed. At each sampling instant, the approximate discrete-time model (DTM) is utilized for solving the optimal control problem online, and the control input applied to continuous-time systems can then be determined. Such RAMPC scheme enables to implement MPC for the continuous-time systems in the digital environment, and meanwhile, achieves the state and control input constraints satisfaction in continuous-time sense. Furthermore, we also provide a guideline to determine the allowable sampling period. Sufficient conditions for the feasibility of the RAMPC scheme as well as the associated stability are developed. Finally, the effectiveness of the RAMPC scheme is shown through a numerical simulation.
Conference Articles
-
A Human-Machine Trust Model Integrating Machine Estimated Performance
Shaojun Chen,
Yun-Bo Zhao ,
Yang Wang,
and Junsen Lu
In 2023 6th International Symposium on Autonomous Systems (ISAS)
2023
[Abs]
[doi]
[pdf]
The prediction of human trust in machines within decision-aid systems is crucial for improving system performance. However, previous studies have only measured machine performance based on its decision history, failing to account for the machine’s current decision state. This delay in evaluating machine performance can result in biased trust predictions, making it challenging to enhance the overall performance of the human-machine system. To address this issue, this paper proposes incorporating machine estimated performance scores into a human-machine trust prediction model to improve trust prediction accuracy and system performance. We also provide an explanation for how this model can enhance system performance. To estimate the accuracy of the machine’s current decision, we employ the KNN method and obtain a corresponding performance score. Next, we report the estimated score to humans through the human-machine interaction interface and obtain human trust via trust self-reporting. Finally, we fit the trust prediction model parameters using data and evaluate the model’s efficacy through simulation on a public dataset. Our ablation experiments show that the model reduces trust prediction bias by 3.6% and significantly enhances the overall accuracy of humanmachine decision-making.
-
Spectrally Normalized Adaptive Neural Identifier for Dynamic Modeling and Trajectory Tracking Control of Unmanned Aerial Vehicle
Shaofeng Chen,
Yu Kang,
Yunbo Zhao,
and Yang Cao
In Advances in Guidance, Navigation and Control
2023
[Abs]
[doi]
[pdf]
Accurate dynamic modeling is difficult for aerobatic unmanned aerial vehicles flying at their physical limit, due to the model uncertainty caused by unobservable hidden states like airflow and vibrations. Although some progresses have been made, these hidden states are still not properly characterized, rendering system identification problem for aerobatic unmanned aerial vehicle extremely challenging. To address this issue, a novel spectrally normalized adaptive neural identifier is proposed for the dynamic modeling of aerobatic unmanned aerial vehicles. Specifically, to characterize the model uncertainty, we propose a spectrally normalized adaptive neural network (SNANet) to extract deep features representing the hidden states of the system. Particularly, the proposed SNANet adopts a multi-model adaptive structure, quickly and dynamically updating the model online. Furthermore, the spectral normalization constraint is introduced into the training process to ensure the Lipschitz stability of the SNANet. Consequently, a trajectory tracking control scheme including the sliding mode controller and SNANet is presented. The modeling effectiveness of the proposed method is verified on a real flight dataset. The results demonstrate that our method has high modeling accuracy, short training time, and fast model response speed.
-
Swap Softmax Twin Delayed Deep Deterministic Policy Gradient
Chaohu Liu,
and Yunbo Zhao
In 2023 6th International Symposium on Autonomous Systems (ISAS)
2023
[Abs]
[doi]
[pdf]
Reinforcement learning algorithms have achieved remarkable success in the realm of continuous control. Among the extensively used algorithms, the Deep Deterministic Policy Gradient algorithm (DDPG) is one of the classic continuous control algorithms, which is prone to the problem of overestimation. Subsequently, the Twin Delayed Deep Deterministic Policy Gradient algorithm (TD3) was proposed, which incorporated the idea of double DQN by taking the minimum value between a pair of critics in order to limit overestimation. Nevertheless, TD3 may lead to an underestimation bias. In order to reduce the effect of errors, we introduce a new method by incorporating Swap Softmax to TD3, which can offset the maximum and minimum values. We evaluate our method on continuous control tasks from OpenAI Gym simulated by MuJoCo and the results show that it has an improvement in performance and robustness.
-
Shared Autonomy Based on Human-in-the-loop Reinforcement Learning with Policy Constraints
Ming Li,
Yu Kang,
Yun-Bo Zhao ,
Jin Zhu,
and Shiyi You
In 2022 41st Chinese Control Conference (CCC)
2022
[Abs]
[doi]
[pdf]
In shared autonomous systems, humans and agents cooperate to complete tasks. Since reinforcement learning enables agents to train good policies through trial and error without knowing the dynamic model of the environment, it has been well applied in shared autonomous systems. After inferring the target from human inputs, agents trained by RL can accurately act accordingly. However, existing methods of this kind have big problems: the training of reinforcement learning algorithms require lots of exploration, which is time-consuming, lack of security guarantee and likely to cause great losses in the training process. Moreover, most of shared control methods are human-oriented, and do not consider the situation that humans may make wrong actions. In view of the above problems, this paper proposes human-in-the-loop reinforcement learning with policy constraints. In the training process, human prior knowledge is used to constrain the exploration of agents to achieve fast and efficient learning. In the process of testing we incorporate policy constraints in the arbitration to avoid serious consequences caused by human mistakes.
-
Strategy Generation Based on DDPG with Prioritized Experience Replay for UCAV
Junsen Lu,
Yun-Bo Zhao ,
Yu Kang,
Yuhui Wang,
and Yimin Deng
In 2022 International Conference on Advanced Robotics and Mechatronics (ICARM)
2022
[Abs]
[doi]
[pdf]
Unmanned combat aerial vehicles are becoming essential participants in future air-combat scenarios, while the optimal control strategy remains a great challenge due to the high dynamics of the aerial vehicles themselves as well as the environmental uncertainties in air-combat. Based on a deep deterministic policy gradient algorithm framework, an air combat decision-making strategy is designed and implemented, and further a prioritized experience replay method is proposed for the proposed algorithm to further improve the efficiency in the training process. Simulation experiments show that, at much reduced training cost, the proposed approach achieves superior air combat performance with fast convergence.
-
Air Combat Maneuver Decision Based on Deep Reinforcement Learning and Game Theory
Shuhui Yin,
Yu Kang,
Yun-Bo Zhao ,
and Jian Xue
In 2022 41st Chinese Control Conference (CCC)
2022
[Abs]
[doi]
[pdf]
The autonomous maneuver decision of UA V plays an important role in future air combat. However, the strong competitiveness of the air combat environment and the uncertainty of the opponent make it difficult to solve the optimal strategy. For these problems, we propose the algorithm based on deep reinforcement learning and game theory, which settles the matter that the existing methods cannot solve Nash equilibrium strategy in highly competitive environment. Specifically, 1 vl air combat is modeled as a two-player zero-sum Markov game, and a simplified two-dimensional simulation environment is constructed. We prove that the algorithm has good convergence through the simulation test. Compared with the opponent’s strategy using DQN, our algorithm has better air combat performance and is more suitable for the air combat game environment.
-
Self-Triggered Model Predictive Control for Perturbed Nonlinear Systems: An Iterative Implementation
Tao Wang,
Pengfei Li,
Yu Kang,
and Yun-Bo Zhao
In 2021 60th IEEE Conference on Decision and Control (CDC)
2021
[Abs]
[doi]
[pdf]
In this paper, a novel iterative self-triggered model predictive control strategy is proposed for continuous-time nonlinear systems with external disturbance. For this strategy, the triggering instants are determined by iteratively using the self-triggered mechanism. To be specific, the triggering mechanism, on the one hand, determines the next sampling instants of the sensor by a prespecified condition, and, on the other hand, decides whether or not to treat the current sampling instant as the triggering instant. Without continuous monitoring of the state, the sensing cost of the sensor can be alleviated. The utilization of the sampling states after the triggering instant leads to a larger triggering interval, and the computational load of the controller can thus be reduced. The effectiveness of the proposed strategy is validated by a numerical example.
-
Approximation-Based Self-Triggered Model Predictive Control for Perturbed Nonlinear Systems
Chang Xu,
Yu Kang,
Yun-Bo Zhao ,
Pengfei Li,
and Tao Wang
In 2021 China Automation Congress (CAC)
2021
[Abs]
[doi]
[pdf]
This paper proposes an approximation-based selftriggered model predictive control strategy for perturbed constrained nonlinear sampled-data systems. In our proposed strategy, the finite horizon optimal control problem (FHOCP) and the triggering condition are designed based on approximate discrete-time models. By implementing the strategy, the computation problem of the FHOCP becomes tractable since it is computed in a discrete-time framework. Meanwhile, the next triggering instant is pre-determined by the triggering condition, reducing the sensing cost and the computing frequency of the FHOCP. Furthermore, feasibility of the FHOCP and stability of the overall system are analyzed. Finally, a simulation example verifies the effectiveness of the strategy.
-
Adaptive Arbitration for Minimal Intervention Shared Control via Deep Reinforcement Learning
Shiyi You,
Yu Kang,
Yun-Bo Zhao ,
and Qianqian Zhang
In 2021 China Automation Congress (CAC)
2021
[Abs]
[doi]
[pdf]
In shared control, humans and intelligent robots jointly complete real-time control tasks with their complementary capabilities for improved performance unavailable by neither side on its own, which is attracting more and more attentions in recent years. Arbitration, as an indispensable part of shared control, determines how control authority is allocated between the human and robot, and the definition of that policy has always been one of the fundamental problems. In this paper, we propose an adaptive arbitration method for shared control systems, which minimizes the deviation from the human inputs while ensuring the system performance based on deep reinforcement learning. We provide humans the maximum assistance with the minimal intervention, in order to balance human’s need for control authority and need for performance. We apply our method to real-time control tasks, and the results show that our method achieves high task success rate and shorter task completion time with less human workload, while maintaining higher human satisfaction.
-
Event-Triggered Adaptive Horizon Model Predictive Control for Perturbed Nonlinear Systems
Pengfei Li,
Tao Wang,
Yu Kang,
and Yun-Bo Zhao
In 2020 59th IEEE Conference on Decision and Control (CDC)
2020
[Abs]
[doi]
[pdf]
This paper proposes a new event-triggered adaptive horizon model predictive control (MPC) for discrete-time nonlinear systems with additive disturbance. With the eventtriggered control scheme, the MPC is solved only at triggering instant and the event is triggered if the difference between the actual state and the predicted state exceeds the triggering threshold. The triggering threshold depends on the prediction horizon and becomes larger as the state approaches the terminal constraint set. Therefore, larger triggering intervals can then be obtained. Finally, a numerical example shows the effectiveness of the proposed scheme.
-
Synthesis of Wireless Networked Control System Based on Round-trip Delay Online Estimation
Liang Lu,
Qipeng Liang,
Qiaohui Zhu,
and Yun-Bo Zhao
In 2020 Chinese Automation Congress (CAC)
2020
[Abs]
[doi]
[pdf]
A control design approach with the integration of online delay estimation is proposed for wireless networked control systems (WNCSs) with unknown round-trip delay, which improves control performance in a practically feasible way. We introduce a delay probability estimation unit to obtain the delay characteristics by estimating the delay when the control system is running. We also present a piecewise approximation control strategy to take advantage of the estimation. Furthermore, the control gain is synthesized with stability guarantee. The conditions to ensure the stochastic stability of the closed-loop system are given, and the effectiveness of the proposed approach is verified numerically.
-
Detection of Distracted Driving Based on Multi-Granularity and Middle-Level Features
Min Tang,
Fang Wu,
Li-Li Zhao,
Qi-Peng Liang,
Jian-Wu Lin,
and Yun-Bo Zhao
In 2020 Chinese Automation Congress (CAC)
2020
[Abs]
[doi]
[pdf]
A so-called MGMN (Multiple-GranularityMiddle Network) algorithm is proposed to improve the detection accuracy of distracted driving, based on multigranularity features and middle-level features. The algorithm is derived from the ResNet50 neural network and is the first time to use multi-granularity features and mid-level features of images in the field of distracted driving detection. The multigranularity feature extraction module consists of three branches: the global branch to learn the global features without local information, the second branch to divide the image level into two parts and later to learn the local features of the upper and lower parts, and the third branch to divide the image level into three parts, and later to learn the local features of the upper, middle and lower parts. By extracting the features of the middle layer of the image, the feature extraction of the algorithm is enriched. While the multi-granularity features are individually input to the cross-entropy loss function, the multi-granularity features of the image and the middle-level features are combined and input into the cross-entropy loss function. The proposed algorithm has an accuracy of 99.8% on the dataset "Districted-DriverDetection" published by State Farm Company, which is 1.5% to 3% higher than existing algorithms, and an improved accuracy of 98.7% on the dataset "AUC-Districted-Driver-Detection".
-
Autonomous Boundary of Human-Machine Collaboration System Based on Reinforcement Learning
Qianqian Zhang,
Yun-Bo Zhao ,
and Yu Kang
In 2020 Australian and New Zealand Control Conference (ANZCC)
2020
[Abs]
[doi]
[pdf]
This paper provides a human-machine collaborative control framework, including artificial intelligence decision systems, human-level control, arbiter judgment, and learning of autonomous boundary, so that human suggestions are incorporated into the training process of decisions, assisting agents to learn quickly control decision tasks. Based on the model-free deep reinforcement learning algorithm HITL-AC, the human feedback (reward or punishment) is connected with the reward of the agent, so that the agent continuously tries to find a better boundary during the system’s operation, avoiding defects of pre-fixed boundary. This formulation improves the data efficiency of reinforcement learning and plays a guiding role in seeking human intervention when the agent is in an uncertain environmental state during the test use phase. The fourth section of the paper gives a training demonstration of the bipedal walker. The experimental results show that human intervention can accelerate the process of agent reinforcement learning during the training phase, and seek human help when guiding the dangerous state of the agent during the test phase. This is beneficial for solving real-world problems, further proving the feasibility and effectiveness of the proposed framework and method.
Books
-
人机混合智能系统自主性理论和方法
赵云波,
康宇,
and 朱进
科学出版社
2021
[Abs]
[pdf]
人工智能(Artificial Intelligence, AI)技术的迅猛发展可能是科技领域近些年最为令人激动人心的现象之一。很少有一项具体的技术引发社会如此广泛的关注,除了技术专家,哲学、法律、道德、社会、管理、经济等各领域的专家学者都在其中各执一词,普通民众也同样表现出极大的热情,莫衷一是。这充分表现出AI技术对我们整个世界方方面面的巨大影响力,也预示着这一技术重塑我们整个世界的巨大潜力。 而谈及未来,一个与我们每个人都息息相关的话题就摆上桌面。在AI从业者那里,这一话题事关所谓的“强弱人工智能之辩”,其中的关键疑问是:现有的仅在某些方面强于人类的“专用人工智能”是否在未来会进化到在所有方面都强于人的“通用人工智能”?而在广大的民众那里,这一强弱人工智能之辩则关乎个人福祉甚至人类命运和尊严:高度发展的专用人工智能可以让创造性含量少的工作丢掉前景,而通用人工智能则更进一步会让整个人类失掉存在价值。这一话题早已在报刊、网络、电视各种公共舆论空间引发广泛的讨论,在真正的未来到来之前,激烈的讨论也远不会有消失的可能。 我们在此无需对这一充满科幻感的话题抛出我们的观点:从目前的技术发展现状来说,这一话题的讨论更多的出于信念,而非依据坚实的技术细节可以做出的可信技术展望。我们只强调如下的事实:AI技术的发展使得由其赋能的机器越来越具有更强大的智能自主能力,并且在越来越多的领域得到了应用,在可预见的未来AI技术的应用似乎还没有减速的迹象。 这一事实把一个原本并不存在或至少并不重要的问题推到了我们面前:在未来的世界里,人的智能和AI赋能的机器智能将无处不在的共存共生,如何在二者之间进行有效融合将成为科学研究的一个重要主题。 人的智能和机器智能在未来的融合共生正是本书的关注点,但本书的主题将更为具体的局限于自动化控制相关的技术领域中。我们认为,人的智能和机器智能在自动化控制领域的共融共存导致了所谓的“人机混合智能系统”的出现,这一新型的系统形式和智能形式在两方面具有本质的重要性:一方面,从自动化控制角度来说,人机混合智能系统所代表的系统结构形式是传统自动化控制系统应对AI赋能的机器智能变革的必然发展形式;另一方面,从智能科学的角度来说,人机混合智能系统所代表的智能形式也成为人工智能未来发展的重要甚至是唯一的终极形式。这两方面本质上的重要性使得建立相关领域的理论和方法框架变得极为急迫和重要。 在本书中,我们试图抛砖引玉,对这一全新而重要的研究领域提供虽然初步但仍然是系统的思考。我们并不希望过多执着于个人的自尊,浅薄地认为本书所提出的理论和方法是面向这一全新领域的必由之路;我们最大的愿望,不过是借由此书,谦卑地展示这一前景广阔而意义重大的研究领域,吸引更多的年轻学者投身其中。
patent
-
一种基于POMDP和面部行为分析的驾驶培训辅助方法
赵云波,
吴芳,
赵丽丽,
and 崔奇
2024
[Abs]
[pdf]
本发明公开了一种基于POMDP和面部行为分析的驾驶培训辅助系统,包括步骤:实时采集学员的头部姿态、脸部表情以及眼睛注视图像,并对采集的图像进行处理;根据得到头部姿态、脸部表情和眼睛注视的图像,估计和预测学员的注意力情况;根据预测的学员的注意力情况和学员的操作结果给予学员适当的驾驶培训辅助提醒或控制。由于人的注意力等内部状态不能被完全直接观察到,只能通过互动和观察来推断,所以这类场景具有不完全可观测性。因此,本文以部分可观马尔可夫决策过程(Partially Observable Markov Decision Process, POMDP)为基础来实现驾驶培训辅助方法。本发明实现了在机器进行驾驶培训辅助的过程中实时地检测人的头部姿态、脸部表情以及眼睛注视,通过对学员的情绪和注意力的判断,基于POMDP实现驾驶辅助的最优决策,给学员提供合适的驾驶辅助输入,帮助学员获得更好的驾驶培训效果。
-
一种基于多算法集成的分歧介入珍珠分拣方法
赵云波,
花婷婷,
赵丽丽,
and 崔奇
2024
[Abs]
[pdf]
一种基于多算法集成的分歧介入珍珠分拣方法,含有:步骤1:将珍珠数据集按类别分别记为Class1-7,并给每张图片制定好标签,按6:2:2的比例分成训练集、验证集和测试集;步骤2:选取目前较为主流先进的三个模型ResNet50、SE-ResNet50和Vgg16进行独立训练,需均能够完成珍珠分拣任务,并分别保存最优模型;步骤3:将获取的三个最优模型用于仲裁系统,即针对构造后的主、次系统给出的预测结果进行分歧仲裁;步骤4:在实验验证阶段,我们使用分歧准确指数和额外成本指数作为系统分歧的评价指标,评估系统的整体性能;步骤5:根据步骤四的评价指标,选取既达到分拣精度提升较高又同时所需的人力成本较少的系统组合作为最终的珍珠分拣系统;步骤6:输出最终分类结果。本发明通过基于多个冗余算法的分歧找出机器分类可能存在的错误预测,并以最少的人力成本介入纠正其预测输出,从而提升整个珍珠分拣的分类精度。
-
一种基于动态信道选择的资源调度方法
赵云波,
and 郝小梅
2022
[Abs]
[pdf]
一种基于动态信道选择的资源调度方法,首先在传感器和控制器之间设计一个信道选择模块,信道选择模块根据系统模型和收到的传感数据来计算得到执行器端上一时刻使用的数据;然后信道选择模块在每个时刻可以比较传感数据和上一个时刻的执行器端使用的数据之间的误差是否小于给定阈值,如果小于给定阈值使用竞争信道传输,大于给定阈值使用非竞争信道传输数据,从而保证每个时刻的执行器端使用的数据与传感数据之间的误差小于给定阈值。最终实现在保证控制系统性能的同时尽量地减少非竞争信道的使用。
-
一种基于多路径切换的无限制DoS攻击防护方法
赵云波,
and 朱巧慧
2022
[Abs]
[pdf]
基于多路径切换的无限制DoS攻击防护方法,首先根据网络丢包率情况和系统模型确定系统可以容忍的最大丢包数,设计DoS攻击检测模块,从而得到多切换路径条件;然后执行器端记录当前传感器到控制器的连续丢包数并发送给传感器,若当前连续丢包数满足路径切换条件,则传感器和控制器切换路径传输数据,若当前连续丢包数没有满足路径切换条件,则继续检测。多路径切换防护方法通过不断检测和切换路径,可以解决无限制DoS攻击造成的连续丢包现象,从而使得系统一直保持稳定。
-
一种基于偏见神经元的数据去偏方法和装置
陈晋音,
陈一鸣,
陈奕芃,
郑海斌,
and 赵云波
[Abs]
本发明公开了一种基于偏见神经元的数据 去偏方法 和装置 ,首先获取原始数 据 ,并 对所述 原始数据中的类别属性进行标记,得到标记后的 数 据集 ,记作数 据集X ;再 翻转数 据集X中的 敏感 属性构成数据集X ′,利用数据集X和数据集X ′筛 选深度学习模型中的偏见神经元;构建反向数据 集;取k的反向数据集中的样本扩充至数据集X中 合成增强数据集,随后将增强数据集输入至深度 模型θ ,并对深度模型θ进行 训练 ,直到深度模 型θ的 分类精度大于80% ,完成去偏。本发明通 过利用深度学习模型中的偏见神经元反向生成 训练 样本 ,将原始样本数据集扩充 增强 ,得到更 加公平的数据集用于深度学习模型的训练,从而 达到消除偏见的目的 ,并且提升深度学习模型决 策的公平性。
-
一种面向深度学习的数据去偏方法及装置
陈晋音,
陈奕芃,
郑海斌,
and 赵云波
[Abs]
本发明公开了一种面向深度学习的数据去 偏方法 及装置 ,首先选取原始数 据集 ,提取原始 数据集中的类别标签以及敏感属性标签;构造判 别模型M1,将原始数据集中的所有样本输入判别 模型M1中 寻找敏感 样本 ,筛选去除 敏感 样本 ,利 用SHAP解释器扩充数 据集 ,得到无偏数 据集 ;构 造预 测模型M2 ,将无偏数据集输入预 测模型M2进 行 训练 ;对 训练得到的 预 测模型M2进行 测试 ,若 满足公平性评估指标机会平等时 ,则认为模型经 训练 后达到公 平 ,完成 去偏 ;若不满足公 平性评 估指标,直至达到公平性评估指标。
-
一种基于深度强化学习的共享自主方法
康宇,
游诗艺,
赵云波,
and 吕文君
[Abs]
本发明公开了一种基于深度强化学习的共 享自 主方法 ,属于人机混合 智能 系统领域。该方 法包括以下步骤:利用长短时记忆网络(LSTM)推 断人类的意图 ;利用深度强化学习训练一个从系 统状态和人类行为到行为奖赏值的端到端映射, 计算每个行为的奖赏值,代表该行为给当前任务 带来的收益;人类行为在其奖赏值下降一定程度 后被判定为无效 ,当人类行为连续多次无效时 , 系统将由机器单独控制,完成由有效行为推断出 的目 标 ,防 止无效行为造成的 危害 ;若人类行为 有效,则利用仲裁函数根据人的行为和机器的计 算结果进行共享控 制 ;根 据上述所有步骤 ,建立 基于深度强化学习的受制于无效人类行为的共 享自治算法。
-
一种基于多粒度特征与中层特征的分心驾驶检测方法
赵云波,
唐敏,
花婷婷,
and 赵丽丽
[Abs]
一种基于多粒度特征与中层特征的分心驾驶检测方法,含有:步骤1:训练融合多粒度特征与中层特征的分心驾驶检测神经网络;步骤2:运用该神经网络对Distracted–Driver–Detection数据集中的数据进行驾驶员注意力集中状况的测试;步骤3:针对步骤2中的测试结果的准确性来调整该神经网络的步长和学习率并再次进行测试直至测试结果的准确率符合条件;步骤4:通过车载终端摄像头实时获取驾驶员的驾驶状态并上传给训练好的该神经网络;步骤5:该神经网络对获取的图片进行识别后在车载终端显示分心驾驶行为。本发明通过车载终端获取驾驶员驾驶状态图像,上传给基于多粒度特征与中层特征的卷积神经网络CNN识别驾驶员分心驾驶行为。
-
一种基于图像的电脑操作员的疲劳检测方法
赵云波,
唐敏,
朱创,
and 孙悦铖
[Abs]
一种基于图像的电脑操作员的疲劳检测方法,含有以下步骤:步骤1:针对需要检测电脑操作员的疲劳状态的场景,拍摄照片构建“电脑操作员”数据集,并按照疲劳状态的类型把照片分类;步骤2:用CNN模型Resnet50模型对自建“电脑操作员”数据集中的训练集部分的数据进行训练,模型根据各张图片中电脑操作员的头部动作和面部特征各个像素点之间的距离关系来确定电脑操作员不同疲劳状态的类型的图片对应的特征;步骤3: 运用CNN模型Resnet50模型对自建“电脑操作员”数据集中的训练集部分的数据进行测试,模型根据步骤2中训练过程提取的图片中电脑操作员不同疲劳状态的类型的特征来判断测试集中给出的新的图片中的电脑操作员属于什么疲劳状态;步骤4:针对步骤3中的测试结果的准确性和来调整CNN模型Resnet50模型中的不同的元素对应的权重和步长的大小并再次进行测试直至测试结果的准确率符合条件; 步骤5:将参数调整后的CNN模型部署到带摄像头的计算机系统中运行;步骤6:通过摄像头实时获取电脑操作员的疲劳状态的类型并输出结果。发明通过布置深度学习环境并且调用电脑摄像头,实时获取电脑操作员的头部动作和面部特征,判断电脑操作员的的疲劳状态。
项目人员
赵云波 刘畅 卢峻森 吴芳 周雅情 夏睿钰 张雯 李明 殷书慧 汪洋 游诗艺 王中月 田霞 花婷婷 蒋舒悦 谢祖浩 赵丽丽 陈少军
项目合作
-
康宇 教授, 中国科学技术大学自动化系
-
朱进 副教授, 中国科学技术大学自动化系
-
陈晋音 教授, 浙江工业大学信息工程学院&网络安全研究院